Machine learning can help doctors diagnose Parkinson's disease by looking at patients' movements
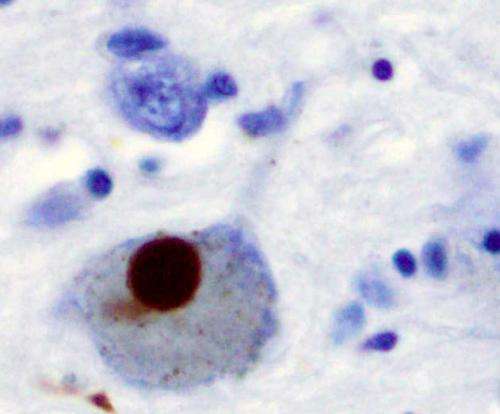
Scientists from Skoltech and A.I. Burnazyan Federal Medical and Biophysical Center have designed and developed a second opinion system based on AI-assisted video analysis that can help medical professionals to objectively assess patients with Parkinson's disease (PD), even at an early stage. This approach can help avoid misdiagnosing this disease, distinguishing between its stages, adjusting therapy and recommending diagnosed patients for deep brain stimulation surgery. The paper was published in IEEE Sensors Journal.
21 feb 2021--A growing number of people with neurodegenerative diseases, due to population aging, will mean that in the coming decades, humanity might face a bona fide Parkinson's disease pandemic. PD, currently the fastest growing neurodegenerative disease, affects the patients' quality of life quite severely and needs to be diagnosed as accurately and as early as possible. The challenge there is to distinguish between Parkinson's and other diseases with similar motor symptoms, for instance, essential tremor. So far, PD has no single biomarker that could be used to diagnose it consistently, and doctors have to rely on their observations, which often lead to wrong diagnoses revealed in pathological examinations.
Assistant Professor Andrey Somov and his colleagues built a so-called second opinion system that uses machine-learning algorithms to analyze video recordings of patients performing specific motor tasks. In a small pilot study, this system showed a very high level of performance in detecting potential cases of PD and distinguishing it from essential tremor.
The system uses video recordings, making the diagnostic process fast, unobtrusive and comfortable for the patients. The team designed a set of 15 common exercises such as walking, sitting down on chair, standing up, folding a towel, filling a glass with water, and touching one's nose with one's index finger. These were general and finer movements, no movement at all (to assess tremor at rest) and some activities that clinicians use to evaluate the tremor.
"The exercises were designed under the supervision of neurologists and came from several different sources, including scales that are used for monitoring Parkinson's disease and previous research done in this area. Each exercise had a target symptom that it could reveal," Ekaterina Kovalenko, Skoltech Ph.D. student and a coauthor of the paper, explained.
In the pilot study, 83 patients with or without neurodegenerative diseases were recorded performing these tasks. The videos were then processed using a piece of software that places keypoints onto the human body corresponding to joints and other parts of the body, creating simplified models of moving subjects. Those were analyzed using machine learning techniques.
The team says that the use of video and machine learning introduces a certain degree of objectiveness into the diagnostic process, allowing researchers and doctors to detect very specific features of the disease and its stages which are not visible to the naked eye.
"Our preliminary results show potential in improving diagnosis with the help of video analysis. Our goal is to provide a second opinion for doctors and clinicians, not to replace them. A video-based method perhaps is the most convenient for patients, as it is the most versatile and noninvasive when compared to various sensors and testing," the authors write in their paper.
"Machine learning and computer vision methods we used in this research are already well established in a number of medical applications; they can be trusted, and the diagnostic exercises for Parkinson's disease have been in development by neurologists for some time. What is truly new about this study is our quantitative ranking of these exercises according to their contribution to a precise and specific final diagnosis. This could only be achieved in collaboration between doctors, mathematicians and engineers," Dmitry Dylov, Skoltech Associate Professor and coauthor of the study, said.
In earlier studies, Somov's team also used wearable sensors in a similar feasibility study that helped them detect the most informative exercises for machine learning-assisted diagnosis of Parkinson's.
"As part of the research process, we had the opportunity to closely interact with doctors and medical personnel, who shared their ideas and experience. It was fascinating observing how two seemingly different disciplines came together to help people. We also had the opportunity to monitor all parts of the research, from designing the methodology to data analysis and machine learning," Kovalenko said.
"This collaboration between doctors and scientists in data analysis allows for many important clinical nuances and details that help achieve the best results. We as doctors see great potential in this; apart from differential diagnosis, we need objective tools to assess motor fluctuation in patients with PD. These tools can provide a more personalized approach to therapy and help make decisions on neurosurgical interventions as well as assess the outcomes of surgery later," neurologist Ekaterina Bril, a coauthor of the paper, noted.
Andrey Somov said the team's next goal is to combine video analysis and sensor data in the task of detecting PD and diagnosing its stages—they expect that this will improve accuracy. "We also keep in mind the innovation aspects of our work—our team agrees that it does make sense to consider converting our research results into an intuitive software product. We believe our joint research efforts will have a positive effect for the patients with PD," he added.
No comments:
Post a Comment